C4W3: Using RNNs to predict time series
C4W3: Using RNNs to predict time series#
import tensorflow as tf
import numpy as np
import matplotlib.pyplot as plt
from dataclasses import dataclass
from tensorflow.keras import layers
def plot_series(time, series, format="-", start=0, end=None):
plt.plot(time[start:end], series[start:end], format)
plt.xlabel("Time")
plt.ylabel("Value")
plt.grid(False)
def trend(time, slope=0):
return slope * time
def seasonal_pattern(season_time):
"""Just an arbitrary pattern, you can change it if you wish"""
return np.where(season_time < 0.1,
np.cos(season_time * 6 * np.pi),
2 / np.exp(9 * season_time))
def seasonality(time, period, amplitude=1, phase=0):
"""Repeats the same pattern at each period"""
season_time = ((time + phase) % period) / period
return amplitude * seasonal_pattern(season_time)
def noise(time, noise_level=1, seed=None):
rnd = np.random.RandomState(seed)
return rnd.randn(len(time)) * noise_level
def generate_time_series():
# The time dimension or the x-coordinate of the time series
time = np.arange(4 * 365 + 1, dtype="float32")
# Initial series is just a straight line with a y-intercept
y_intercept = 10
slope = 0.005
series = trend(time, slope) + y_intercept
# Adding seasonality
amplitude = 50
series += seasonality(time, period=365, amplitude=amplitude)
# Adding some noise
noise_level = 3
series += noise(time, noise_level, seed=51)
return time, series
# Save all "global" variables within the G class (G stands for global)
@dataclass
class G:
TIME, SERIES = generate_time_series()
SPLIT_TIME = 1100
WINDOW_SIZE = 20
BATCH_SIZE = 32
SHUFFLE_BUFFER_SIZE = 1000
# Plot the generated series
plt.figure(figsize=(10, 6))
plot_series(G.TIME, G.SERIES)
plt.show()
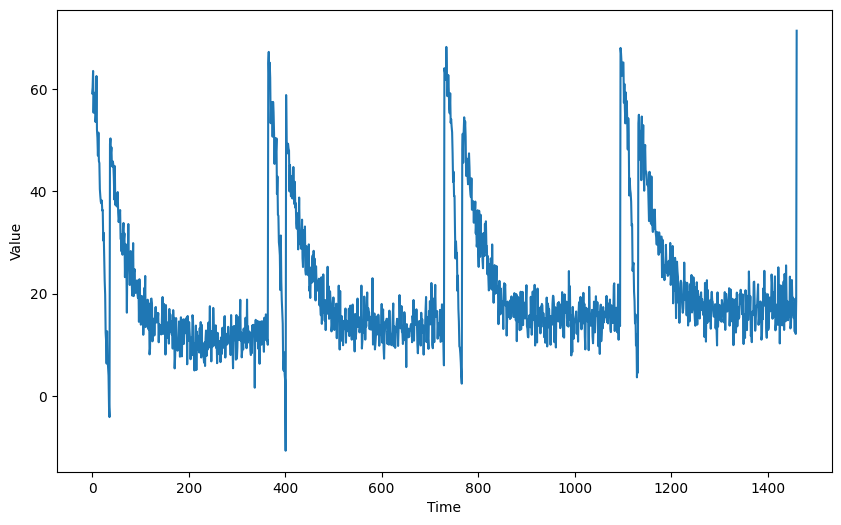
def train_val_split(time, series, time_step=G.SPLIT_TIME):
time_train = time[:time_step]
series_train = series[:time_step]
time_valid = time[time_step:]
series_valid = series[time_step:]
return time_train, series_train, time_valid, series_valid
time_train, series_train, time_valid, series_valid = train_val_split(G.TIME, G.SERIES)
def windowed_dataset(series, window_size=G.WINDOW_SIZE, batch_size=G.BATCH_SIZE, shuffle_buffer=G.SHUFFLE_BUFFER_SIZE):
dataset = tf.data.Dataset.from_tensor_slices(series)
dataset = dataset.window(window_size + 1, shift=1, drop_remainder=True)
dataset = dataset.flat_map(lambda window: window.batch(window_size + 1))
dataset = dataset.shuffle(shuffle_buffer)
dataset = dataset.map(lambda window: (window[:-1], window[-1]))
dataset = dataset.batch(batch_size).prefetch(tf.data.AUTOTUNE)
return dataset
dataset = windowed_dataset(series_train)
def create_uncompiled_model():
model = tf.keras.Sequential([
layers.Lambda(lambda x: tf.expand_dims(x, axis=-1), input_shape=[None]),
layers.Bidirectional(layers.LSTM(128)),
layers.Dense(1),
layers.Lambda(lambda x: x * 100.0)
])
return model
def create_model():
tf.random.set_seed(51)
model = create_uncompiled_model()
model.compile(optimizer='adam',
loss='huber',
metrics=["mae"])
return model
model = create_model()
history = model.fit(dataset, epochs=50)
Epoch 1/50
34/34 [==============================] - 4s 18ms/step - loss: 9.6444 - mae: 10.1342
Epoch 2/50
34/34 [==============================] - 1s 17ms/step - loss: 3.8921 - mae: 4.3643
Epoch 3/50
34/34 [==============================] - 1s 20ms/step - loss: 3.4016 - mae: 3.8729
Epoch 4/50
34/34 [==============================] - 1s 15ms/step - loss: 4.3176 - mae: 4.7990
Epoch 5/50
34/34 [==============================] - 1s 15ms/step - loss: 2.9624 - mae: 3.4270
Epoch 6/50
34/34 [==============================] - 1s 15ms/step - loss: 4.1799 - mae: 4.6543
Epoch 7/50
34/34 [==============================] - 1s 15ms/step - loss: 3.3709 - mae: 3.8420
Epoch 8/50
34/34 [==============================] - 1s 16ms/step - loss: 3.0171 - mae: 3.4820
Epoch 9/50
34/34 [==============================] - 1s 16ms/step - loss: 3.1093 - mae: 3.5768
Epoch 10/50
34/34 [==============================] - 1s 16ms/step - loss: 3.1179 - mae: 3.5856
Epoch 11/50
34/34 [==============================] - 1s 15ms/step - loss: 2.5994 - mae: 3.0639
Epoch 12/50
34/34 [==============================] - 1s 15ms/step - loss: 2.5901 - mae: 3.0502
Epoch 13/50
34/34 [==============================] - 1s 15ms/step - loss: 2.8696 - mae: 3.3315
Epoch 14/50
34/34 [==============================] - 1s 16ms/step - loss: 3.2494 - mae: 3.7174
Epoch 15/50
34/34 [==============================] - 1s 16ms/step - loss: 2.9485 - mae: 3.4159
Epoch 16/50
34/34 [==============================] - 1s 14ms/step - loss: 2.7890 - mae: 3.2580
Epoch 17/50
34/34 [==============================] - 1s 15ms/step - loss: 2.5647 - mae: 3.0247
Epoch 18/50
34/34 [==============================] - 1s 15ms/step - loss: 2.7590 - mae: 3.2284
Epoch 19/50
34/34 [==============================] - 1s 16ms/step - loss: 2.8208 - mae: 3.2837
Epoch 20/50
34/34 [==============================] - 1s 14ms/step - loss: 2.8933 - mae: 3.3608
Epoch 21/50
34/34 [==============================] - 1s 14ms/step - loss: 2.8414 - mae: 3.3048
Epoch 22/50
34/34 [==============================] - 1s 18ms/step - loss: 2.8532 - mae: 3.3182
Epoch 23/50
34/34 [==============================] - 1s 16ms/step - loss: 2.7460 - mae: 3.2093
Epoch 24/50
34/34 [==============================] - 1s 15ms/step - loss: 3.0854 - mae: 3.5556
Epoch 25/50
34/34 [==============================] - 1s 15ms/step - loss: 2.7863 - mae: 3.2493
Epoch 26/50
34/34 [==============================] - 1s 15ms/step - loss: 2.6859 - mae: 3.1538
Epoch 27/50
34/34 [==============================] - 1s 17ms/step - loss: 2.9882 - mae: 3.4599
Epoch 28/50
34/34 [==============================] - 1s 16ms/step - loss: 2.6589 - mae: 3.1222
Epoch 29/50
34/34 [==============================] - 1s 16ms/step - loss: 2.6606 - mae: 3.1236
Epoch 30/50
34/34 [==============================] - 1s 16ms/step - loss: 3.0686 - mae: 3.5392
Epoch 31/50
34/34 [==============================] - 1s 16ms/step - loss: 3.2697 - mae: 3.7444
Epoch 32/50
34/34 [==============================] - 1s 16ms/step - loss: 2.6444 - mae: 3.1040
Epoch 33/50
34/34 [==============================] - 1s 16ms/step - loss: 2.8491 - mae: 3.3129
Epoch 34/50
34/34 [==============================] - 1s 14ms/step - loss: 2.7825 - mae: 3.2511
Epoch 35/50
34/34 [==============================] - 1s 18ms/step - loss: 2.6066 - mae: 3.0676
Epoch 36/50
34/34 [==============================] - 1s 16ms/step - loss: 2.6518 - mae: 3.1175
Epoch 37/50
34/34 [==============================] - 1s 14ms/step - loss: 2.7951 - mae: 3.2597
Epoch 38/50
34/34 [==============================] - 1s 15ms/step - loss: 2.9802 - mae: 3.4519
Epoch 39/50
34/34 [==============================] - 1s 14ms/step - loss: 2.5441 - mae: 3.0073
Epoch 40/50
34/34 [==============================] - 1s 15ms/step - loss: 2.5085 - mae: 2.9691
Epoch 41/50
34/34 [==============================] - 1s 15ms/step - loss: 2.4125 - mae: 2.8691
Epoch 42/50
34/34 [==============================] - 1s 14ms/step - loss: 2.9066 - mae: 3.3802
Epoch 43/50
34/34 [==============================] - 1s 15ms/step - loss: 2.6436 - mae: 3.1020
Epoch 44/50
34/34 [==============================] - 1s 15ms/step - loss: 2.4927 - mae: 2.9495
Epoch 45/50
34/34 [==============================] - 1s 16ms/step - loss: 2.5427 - mae: 3.0068
Epoch 46/50
34/34 [==============================] - 1s 15ms/step - loss: 2.5312 - mae: 2.9906
Epoch 47/50
34/34 [==============================] - 1s 15ms/step - loss: 2.7195 - mae: 3.1796
Epoch 48/50
34/34 [==============================] - 1s 16ms/step - loss: 2.3772 - mae: 2.8390
Epoch 49/50
34/34 [==============================] - 1s 15ms/step - loss: 2.6211 - mae: 3.0817
Epoch 50/50
34/34 [==============================] - 1s 15ms/step - loss: 2.5150 - mae: 2.9682
def compute_metrics(true_series, forecast):
mse = tf.keras.metrics.mean_squared_error(true_series, forecast).numpy()
mae = tf.keras.metrics.mean_absolute_error(true_series, forecast).numpy()
return mse, mae
def model_forecast(model, series, window_size):
ds = tf.data.Dataset.from_tensor_slices(series)
ds = ds.window(window_size, shift=1, drop_remainder=True)
ds = ds.flat_map(lambda w: w.batch(window_size))
ds = ds.batch(32).cache().prefetch(tf.data.AUTOTUNE)
forecast = model.predict(ds)
return forecast
forecast_series = G.SERIES[G.SPLIT_TIME - G.WINDOW_SIZE:-1]
rnn_forecast = model_forecast(model, forecast_series, G.WINDOW_SIZE).squeeze()
plt.figure(figsize=(10, 6))
plot_series(time_valid, series_valid)
plot_series(time_valid, rnn_forecast)
12/12 [==============================] - 1s 10ms/step
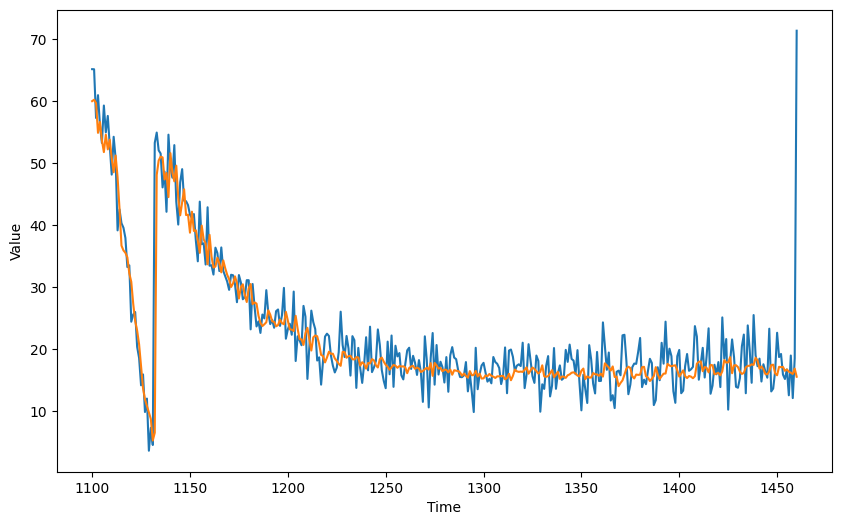
mse, mae = compute_metrics(series_valid, rnn_forecast)
print(f"mse: {mse:.2f}, mae: {mae:.2f} for forecast")
mse: 26.81, mae: 3.06 for forecast
# model.save('my_model')