Best practices for model deployment and serving
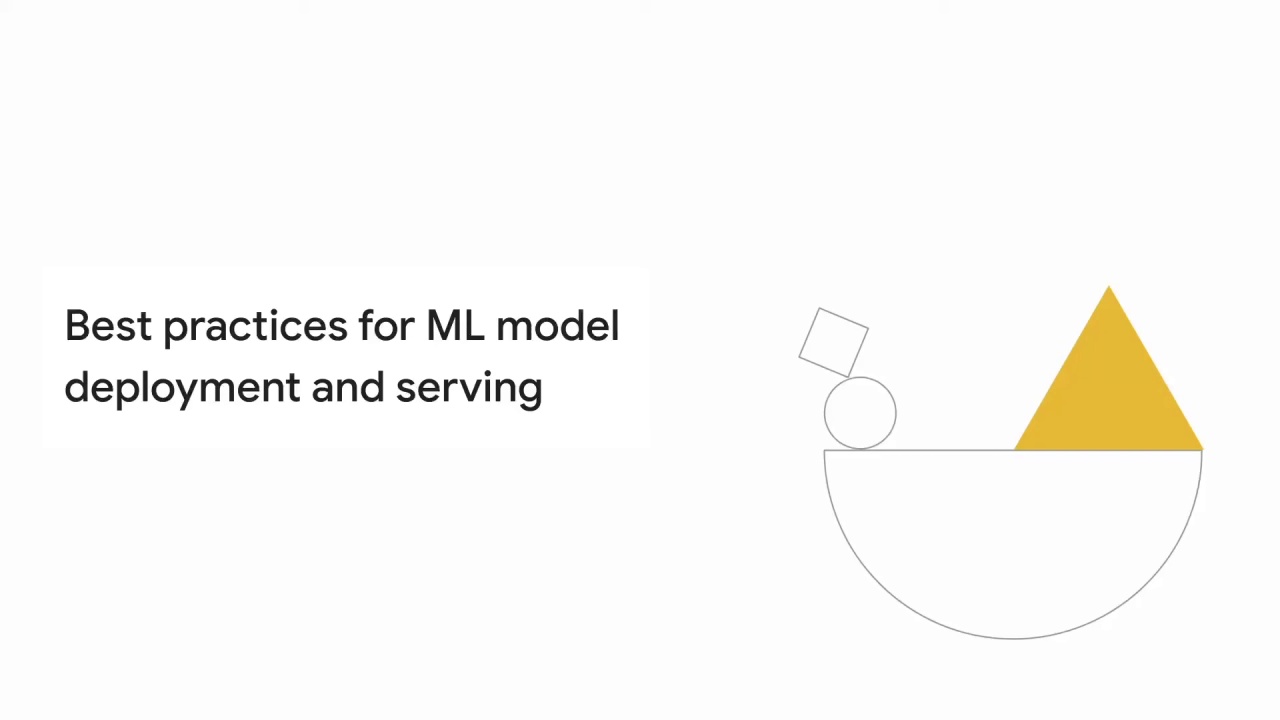
In this lesson, we provide an overview of best practices
for model deployment and serving.
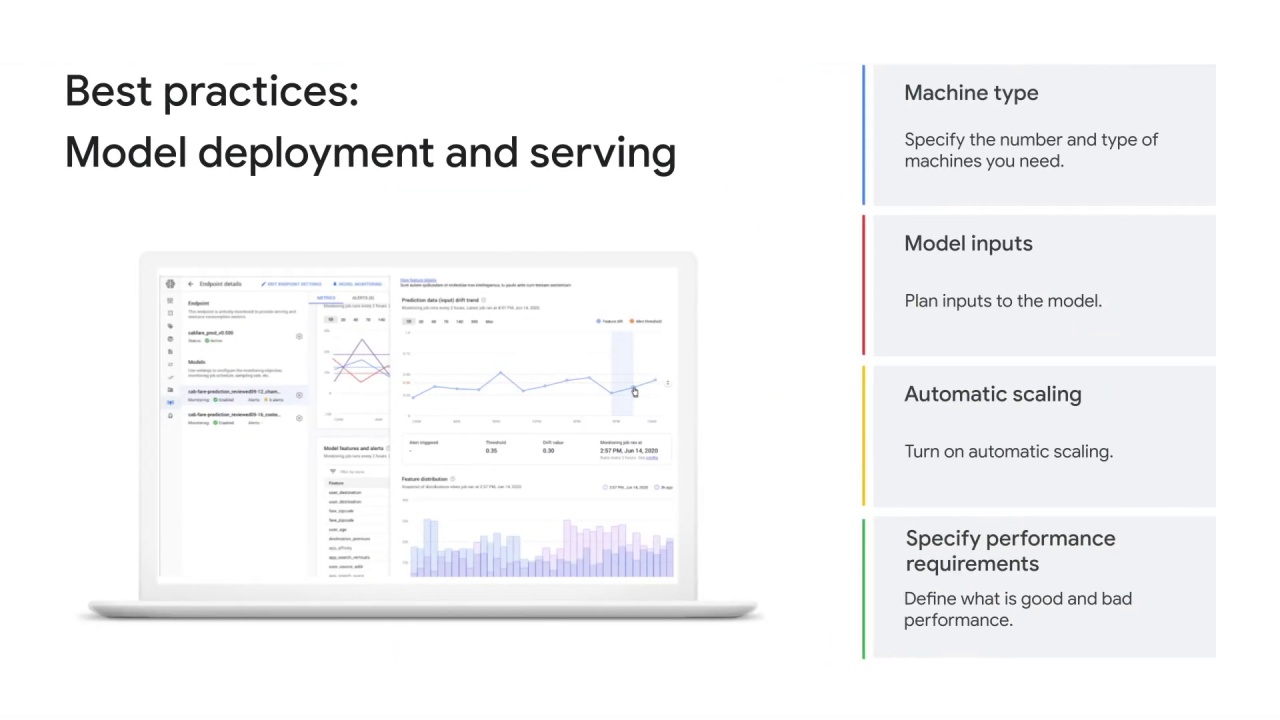
Model deployment and serving refers to putting a model into production.
The output of the training job is a model file stored on Cloud Storage, which you can upload to Vertex AI so the file can be used for prediction serving.
For best practices
for model deployment and serving:
specify the
number and types of machines you need
,plan
inputs to the model
,turn on
automatic scaling
,and define
what is good and bad performance
.
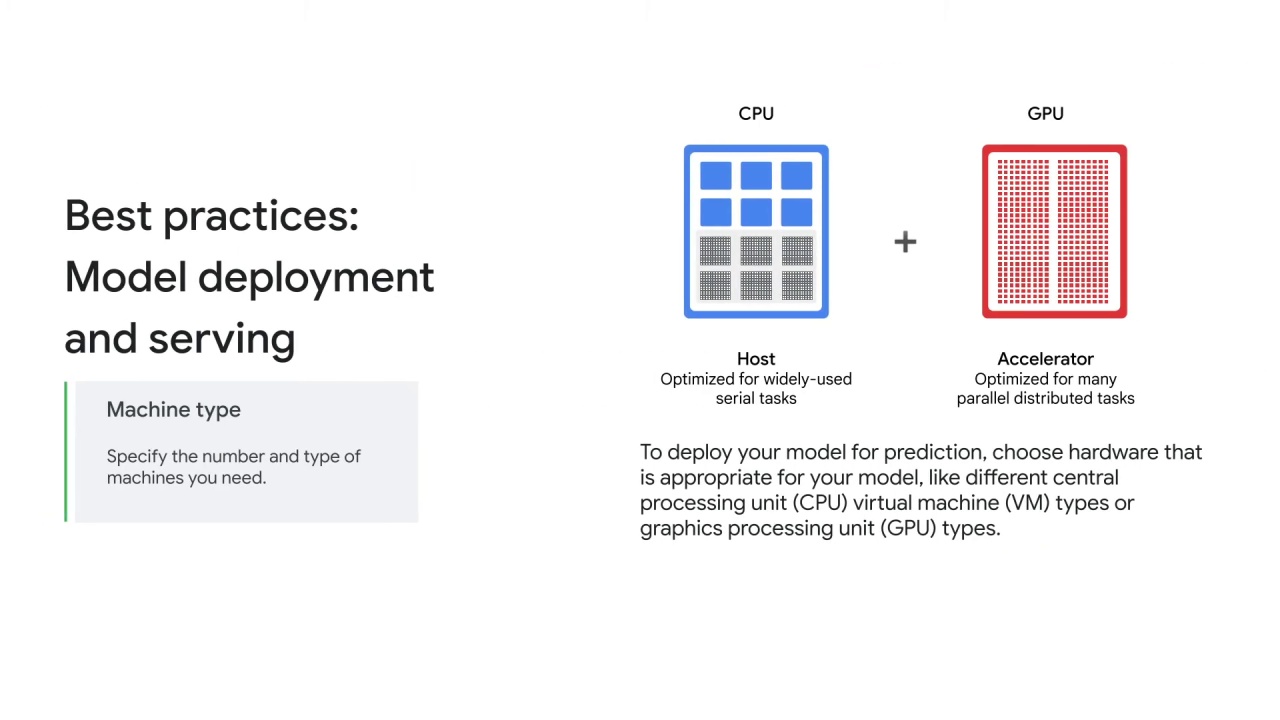
To deploy your model for prediction, choose hardware that’s appropriate for your model, like different:
central processing unit (
CPU
),virtual machine (
VM
) types,or graphics processing unit (
GPU
) types.
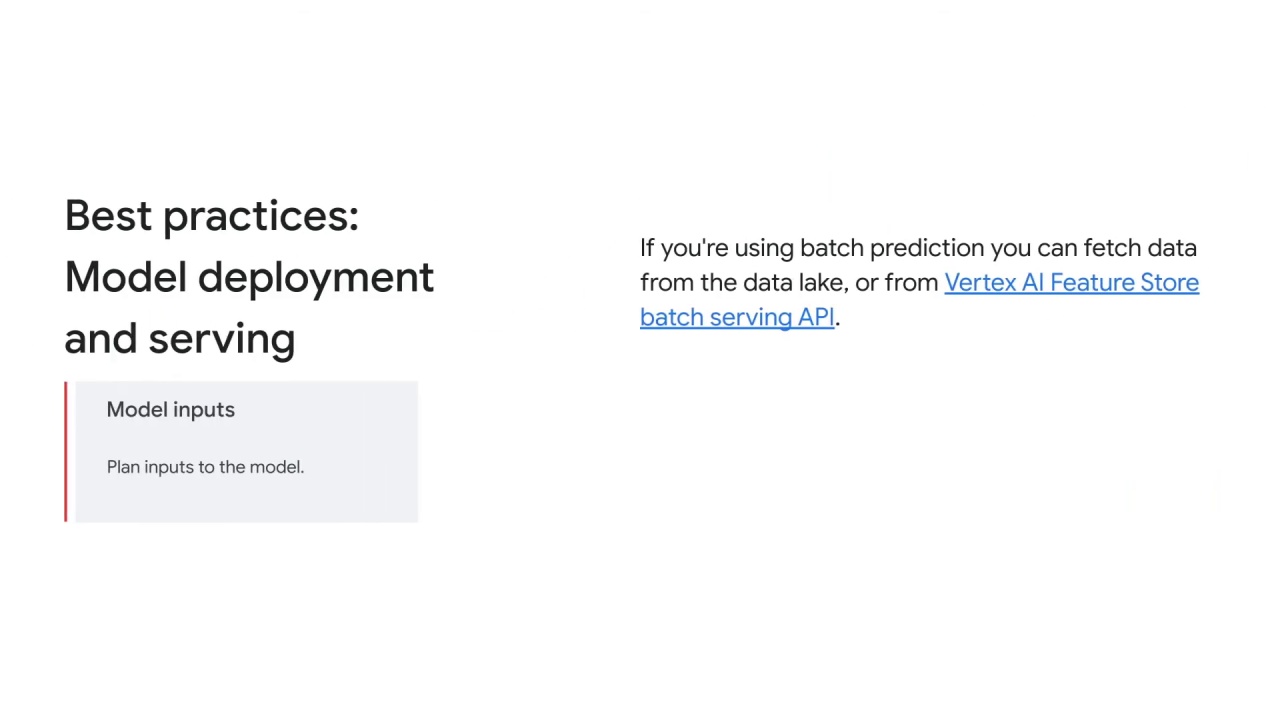
In addition to deploying a model, you’ll need to determine how you’re going to parse inputs
to the model.
If you’re using batch prediction, you can fetch data from the data lake or from Vertex AI Feature Store batch serving API.
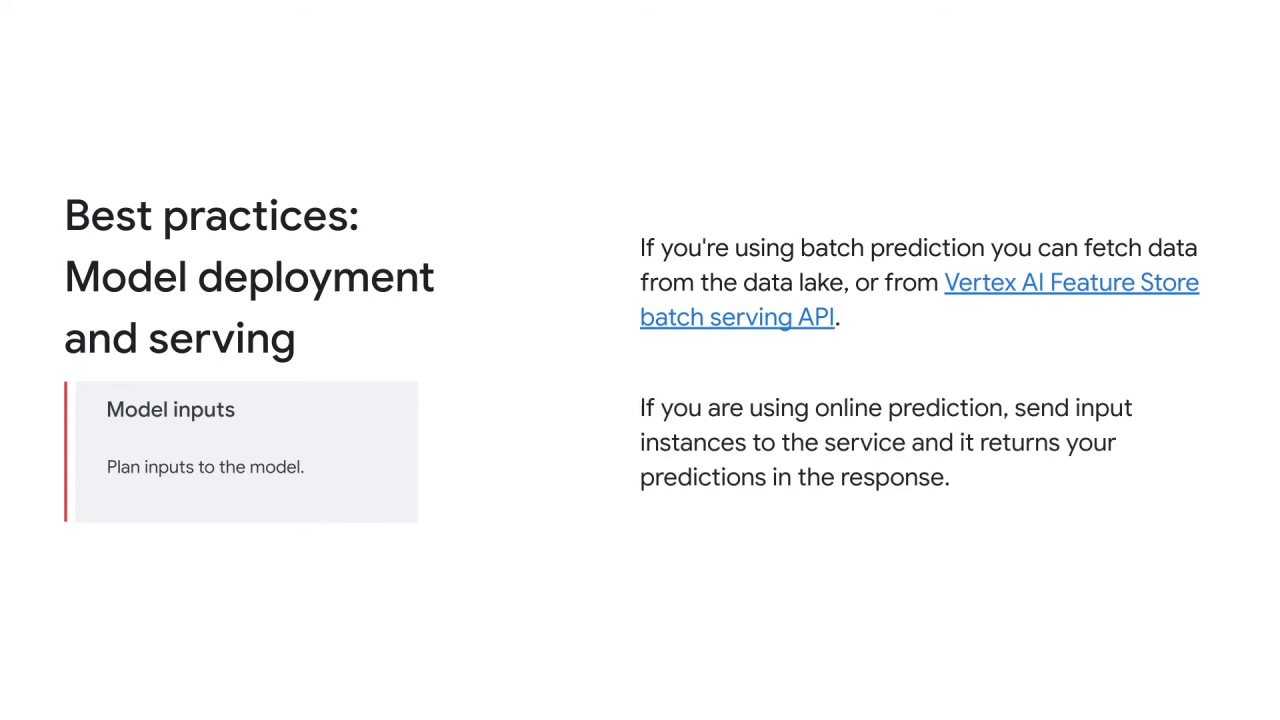
If you’re using online prediction, you can send input instances to the service, and it returns your predictions in the response.
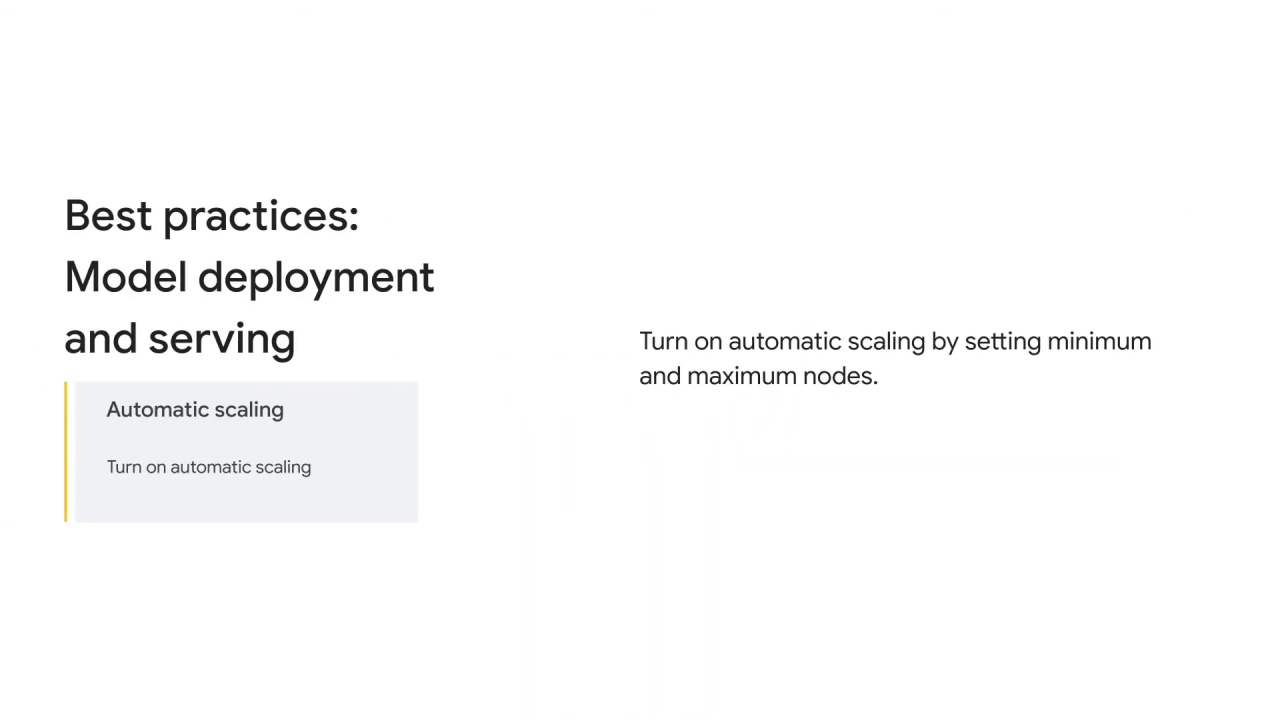
If you use the online prediction service, in most cases, we recommend that you turn on automatic scaling by setting minimum and maximum nodes.
To ensure a high-availability service level agreement (SLA), set automatic scaling with a minimum of two nodes
.
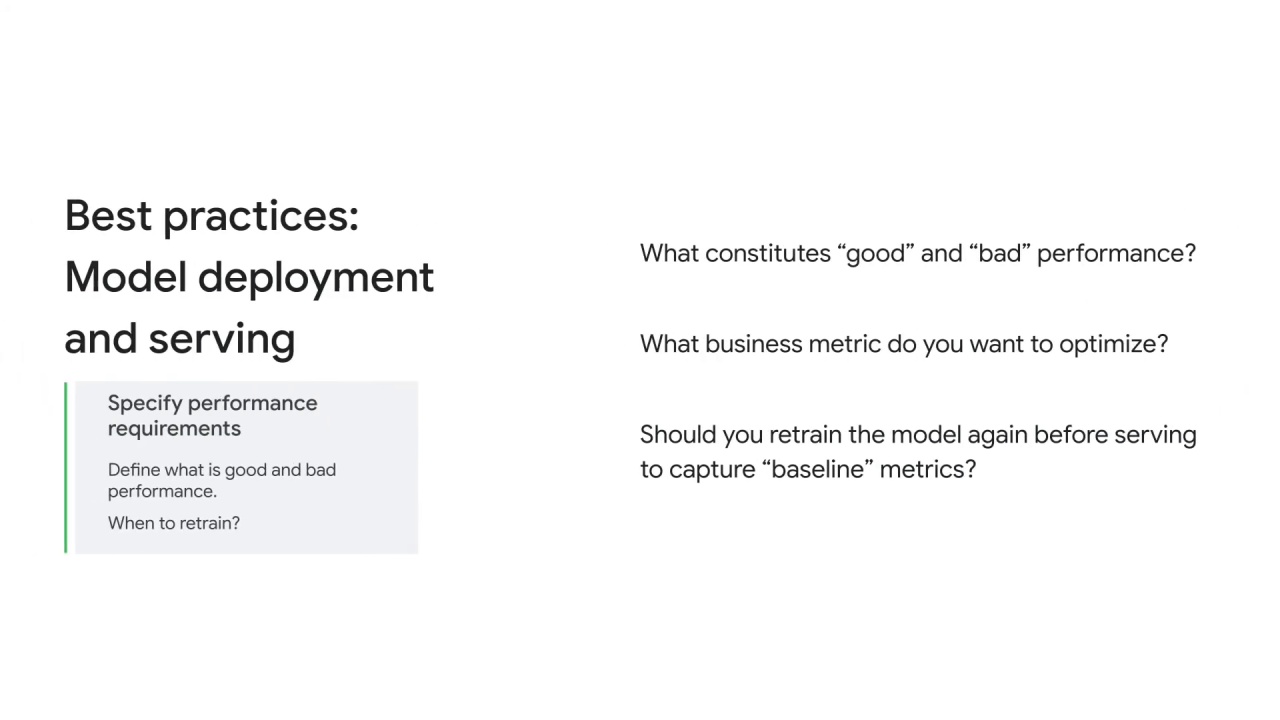
When you specify performance requirements, you’re clarifying what constitutes “good” and “bad” performance.
Recall that the first step
in the ML process is to identify a goal or problem.
How do you specify the performance requirements?
In machine learning, we refer to this as what metric you want to optimize.
Business metrics such as ROC
, AUC
, or RMSE
should match your business objective.
Because a machine learning model’s performance degrades over time in production, you should evaluate retraining requirements before model serving.
Based on the use case, model monitoring, and evaluation, you can decide when to retrain the model again.