Vertex AI pipeline best practices
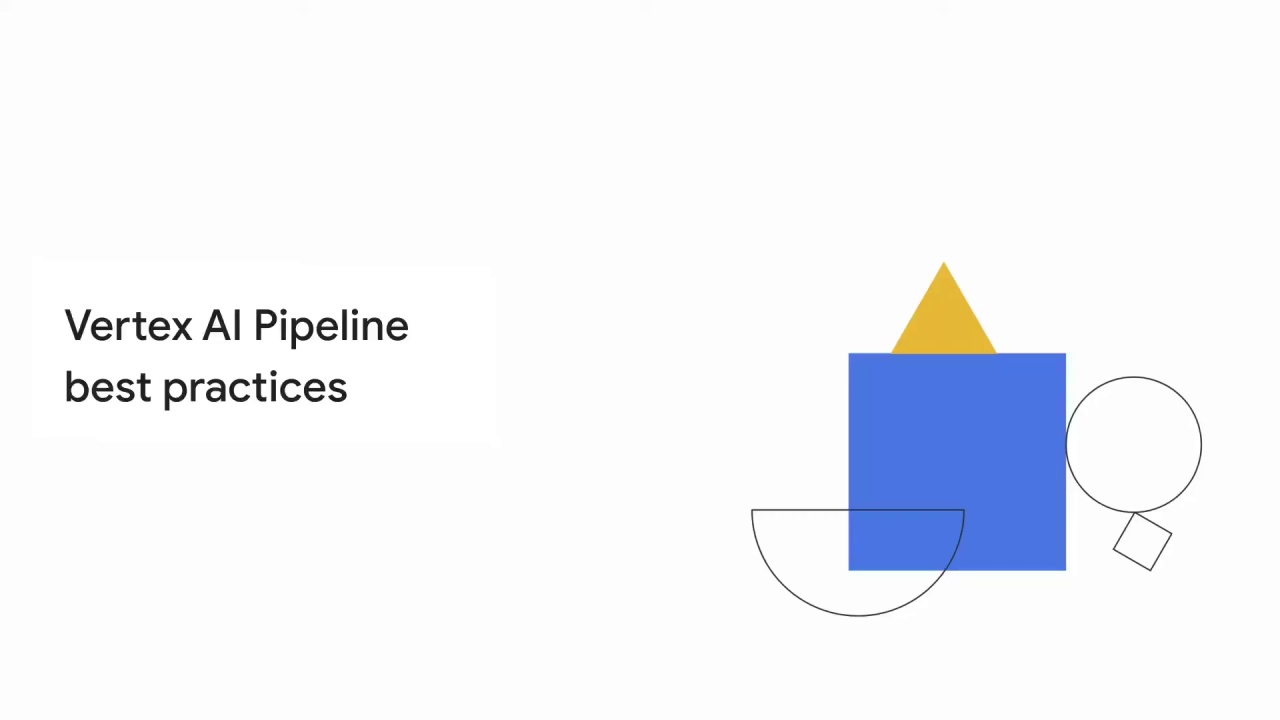
In this lesson, we provide an overview of Vertex AI Pipelines, what they are and how they’re created.
We then provide an overview of best practices
.
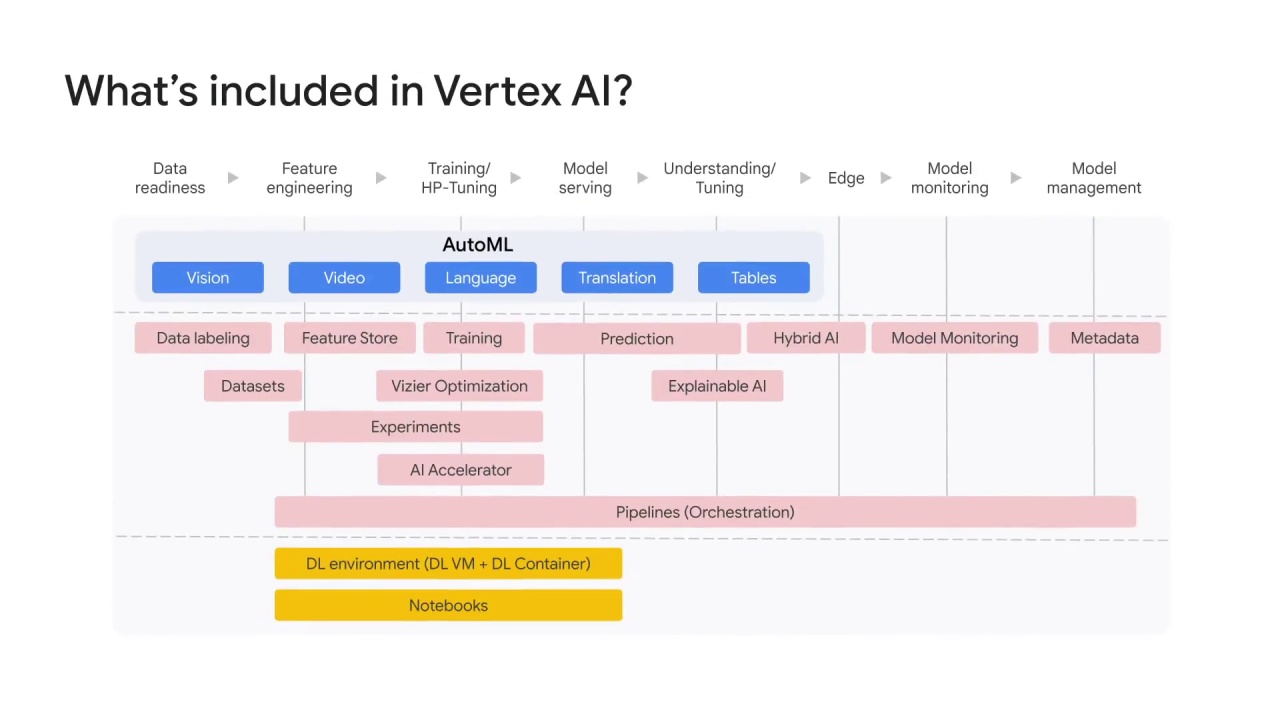
As stated previously, Vertex AI provides a suite of products to help you at each stage of your ML workflow from gathering data to feature engineering to building models and finally, deploying and monitoring those models.
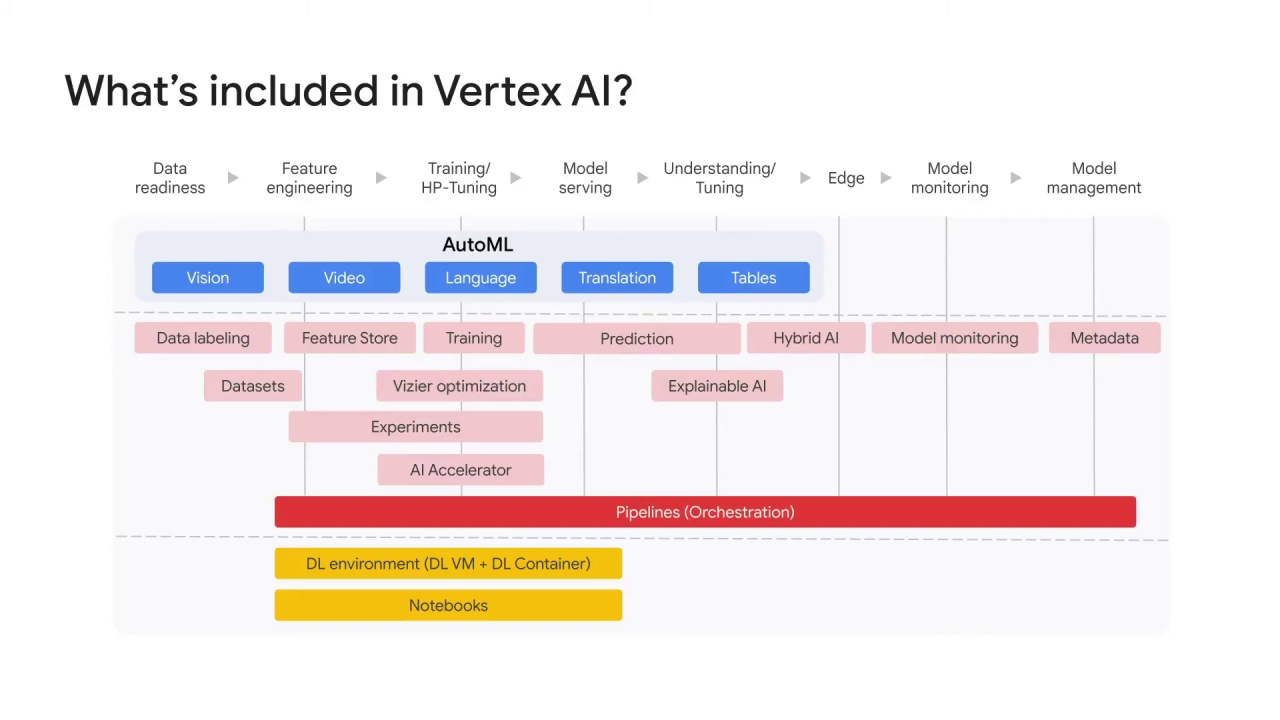
Vertex AI Pipelines helps you to automate, monitor and govern
your ML systems by orchestrating your ML workflow in a serverless
manner.
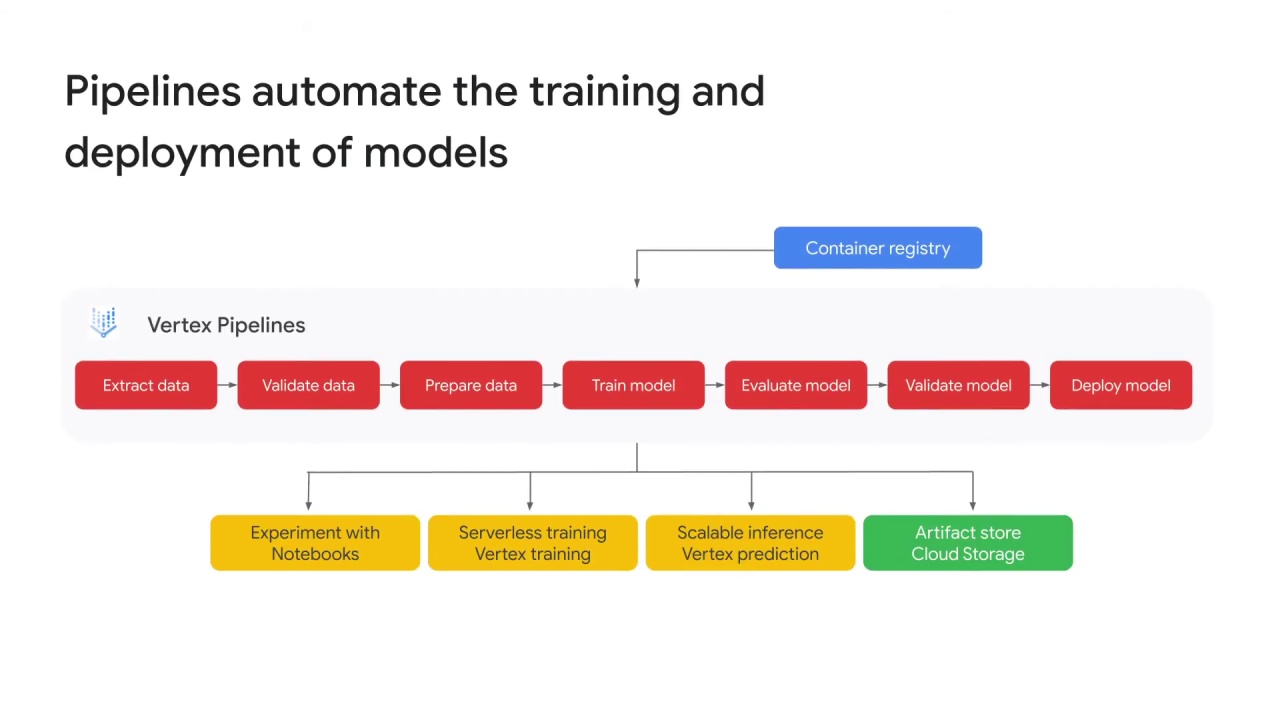
Pipelines automate the training and deployment of models.
This example ML workflow shows the seven components of the ML workflow that encapsulates a Vertex AI Pipeline from data extraction to model deployment.
Note that Vertex AI Services such as Notebooks, Vertex Training, Vertex Prediction and artifact store all benefit from pipeline components.
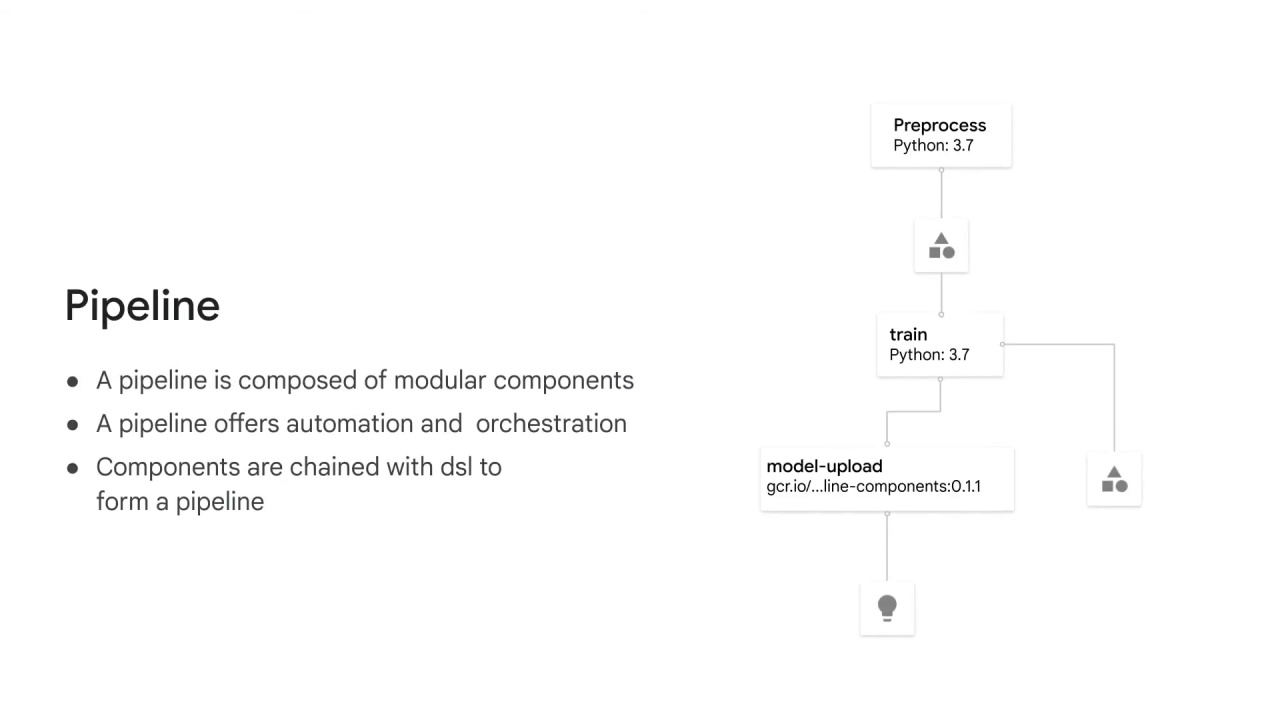
A pipeline is built of modular components and offers automation and orchestration.
Components are chained with DSL, or domain-specific language, to form a pipeline.
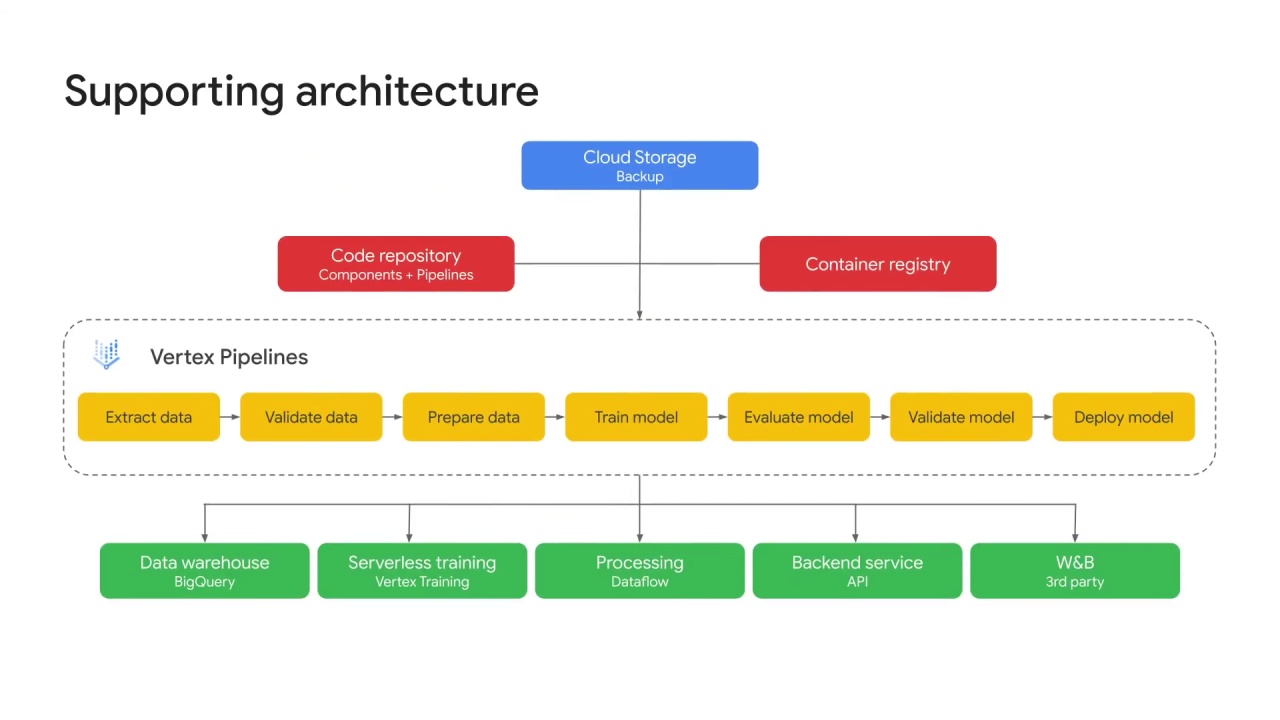
This example shows the supporting architecture of an organization.
Notice that it can feed BigQuery, the Vertex training service, Dataflow and the company’s backend API services.
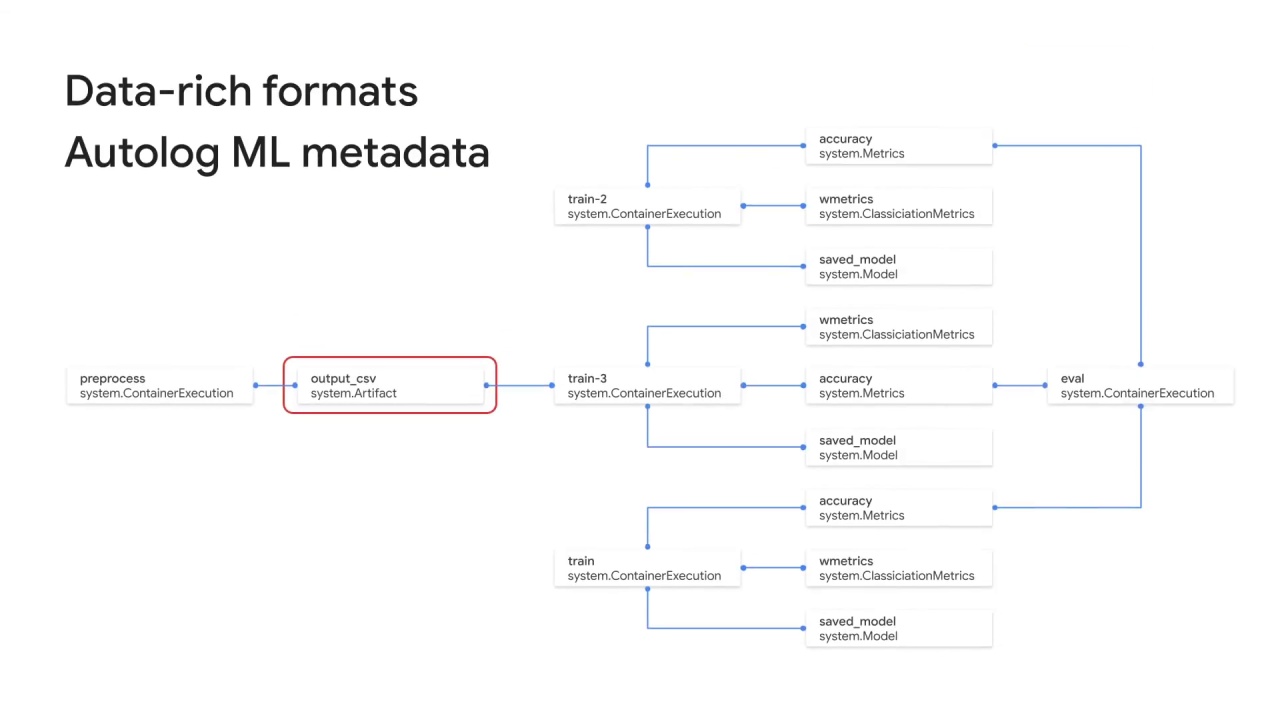
In order to understand changes in the performance or accuracy of your ML system, you must be able to analyze the metadata of pipeline runs and the lineage of ML artifacts.
An artifact lineage includes all the factors that contributed to its creation such as model training, datasets and hyperparameters and artifacts and metadata that are derived from this artifact.
Managing this metadata in an ad hoc manner can be difficult and time-consuming.
Let’s review some best practices
.
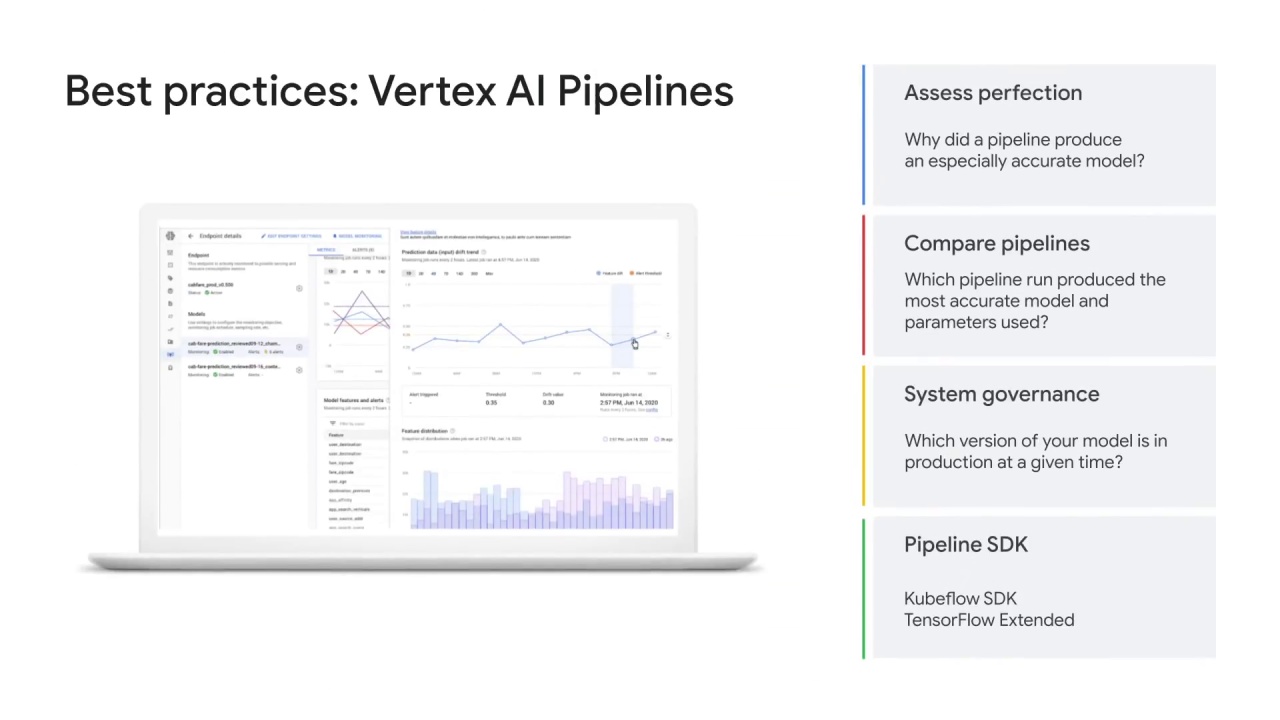
As previously noted, when you run a pipeline using Vertex AI Pipelines, the artifacts and metadata of your pipeline run are stored using Vertex ML Metadata.
As a best practice
, assess perfection.
Why did a certain pipeline run produce an especially accurate model?
Compare pipelines.
Which pipeline run produced the most accurate model, and what type of parameters were used to train the model?
Implement system governance.
Depending on the steps in your pipeline, you may be able to use Vertex ML Metadata to answer system governance questions.
For example, you could use metadata to determine which version of your model was in production at a given point in time.
Vertex AI Pipelines lets you orchestrate your machine learning workflows in a serverless manner.
Before Vertex AI Pipelines can orchestrate your ML workflow, you must describe your workflow as a pipeline.
ML pipelines are portable and scalable ML workflows that are based on containers in Google Cloud Services.
Vertex AI Pipelines can run pipelines built using the Kubeflow Pipelines SDK or TensorFlow Extended.
If you use TensorFlow in an ML workflow that processes terabytes of structured data or text data, we recommend that you build your pipeline using TFX.
For other use cases, we recommend that you build your pipeline using the Kubeflow Pipelines SDK.
With this SDK, you can implement your workflow by building custom components or reusing prebuilt components such as the Google Cloud Pipeline Components.
Google Cloud Pipeline Components make it easier to use Vertex AI services like AutoML in your pipeline.